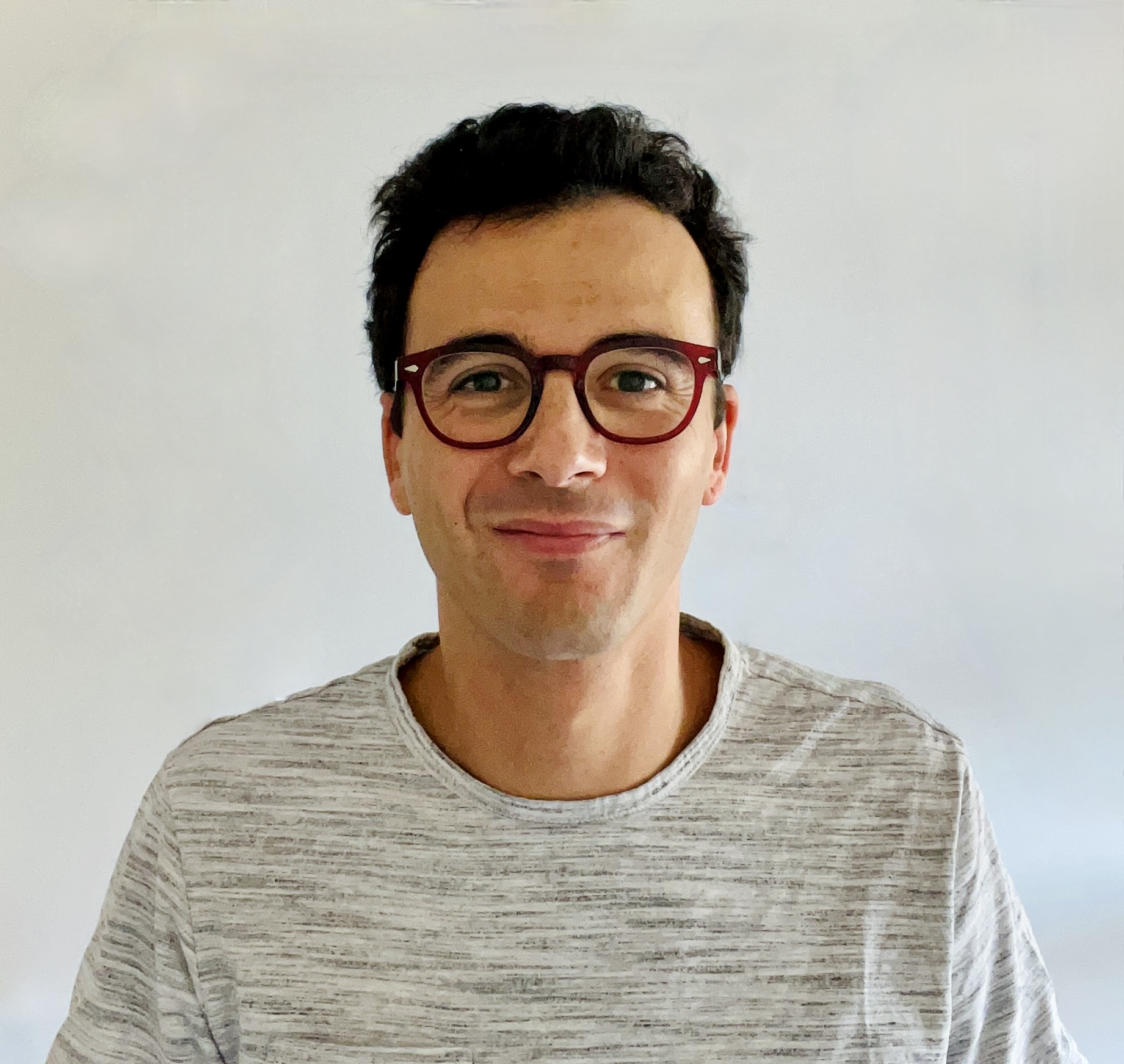
Associate Professor (Reader) in Machine Learning
School of Mathematics, The University of Edinburgh
The Maxwell Institute for Mathematical Sciences
School of Mathematics, Gran Sasso Science Institute
JCMB, King’s Buildings, Edinburgh EH93FD UK
email: f dot tudisco at ed.ac.uk